本研究課題適合遙感、規(guī)劃、地理信息系統(tǒng)等學(xué)科領(lǐng)域的學(xué)生與從業(yè)者
來源:原創(chuàng)?作者:學(xué)姐
編輯:學(xué)姐
CV方向遙感課題研究什么?
大規(guī)模城市土地覆被分類是遙感領(lǐng)域的經(jīng)典課題,它引導(dǎo)著城市基礎(chǔ)設(shè)施規(guī)劃、產(chǎn)業(yè)布局、資源管理等,是政策制定者的重點(diǎn)關(guān)注內(nèi)容之一。
同時,精細(xì)化的城市模型為智慧城市、智慧交通、城市氣候環(huán)境的改善奠定了基礎(chǔ)。
近年來,隨著深度學(xué)習(xí)技術(shù)的發(fā)展,各頭部科技公司與互聯(lián)網(wǎng)企業(yè)也越來越重視城市模型的建設(shè)與投入,將新技術(shù)與算法引入到這一經(jīng)典研究課題中。
例如,華為、商湯等企業(yè)近年來多次舉辦遙感圖像分類與分割的挑戰(zhàn)賽;阿里巴巴啟動了城市大腦項(xiàng)目;騰訊成立了智慧城市事業(yè)部等等……
然而,對于學(xué)術(shù)界而言,高清的商業(yè)遙感衛(wèi)星圖像昂貴且難以獲得,如何通過開源的中低分辨率衛(wèi)星圖像制作高精度的城市分類地圖成為了挑戰(zhàn)。
本課題將聚焦經(jīng)典,完成基于開源遙感數(shù)據(jù)的大規(guī)模城市土地覆被分類實(shí)踐,著重介紹世界城市數(shù)據(jù)庫組織(World Urban Database and Access Portal Tools, WUDAPT)的方法和成果,并完成深度卷積神經(jīng)網(wǎng)絡(luò)的設(shè)計和在城市土地覆蓋分類中的應(yīng)用。
【課題】基于開源遙感數(shù)據(jù)的大規(guī)模城市土地覆被分類——WUDAPT方法與深度學(xué)習(xí)方法的比較
該方向必讀論文如下
[1] Stewart, I.D. and T.R. Oke, Local climate zones for urban temperature studies. Bulletin of the American Meteorological Society, 2012. 93(12): p. 1879-1900.
[2] Bechtel, B., et al., Mapping local climate zones for a worldwide database of the form and function of cities. ISPRS International Journal of Geo-Information, 2015. 4(1): p. 199-219.
[3] Bechtel, B., et al., Towards consistent mapping of urban structure-global human settlement layer and local climate zones. ISPRS-International Archives of the Photogrammetry, Remote Sensing and Spatial Information Sciences, 2016. 41: p. 1371-1378.
[4] Ma, L., Liu, Y., Zhang, X., Ye, Y., Yin, G., & Johnson, B. A. (2019). Deep learning in remote sensing applications: A meta-analysis and review. ISPRS journal of photogrammetry and remote sensing, 152, 166-177.
[5] Li, Y., Zhang, H., Xue, X., Jiang, Y., & Shen, Q. (2018). Deep learning for remote sensing image classification: A survey. Wiley Interdisciplinary Reviews: Data Mining and Knowledge Discovery, 8(6), e1264.
[6] Xu, Y., Ren, C., Cai, M., Edward, N. Y. Y., & Wu, T. (2017). Classification of local climate zones using ASTER and Landsat data for high-density cities. IEEE Journal of Selected Topics in Applied Earth Observations and Remote Sensing, 10(7), 3397-3405.
[7] Chen, F., & Tsou, J. Y. (2021). DRSNet: Novel architecture for small patch and low-resolution remote sensing image scene classification. International Journal of Applied Earth Observation and Geoinformation, 104, 102577.
論文領(lǐng)取方法
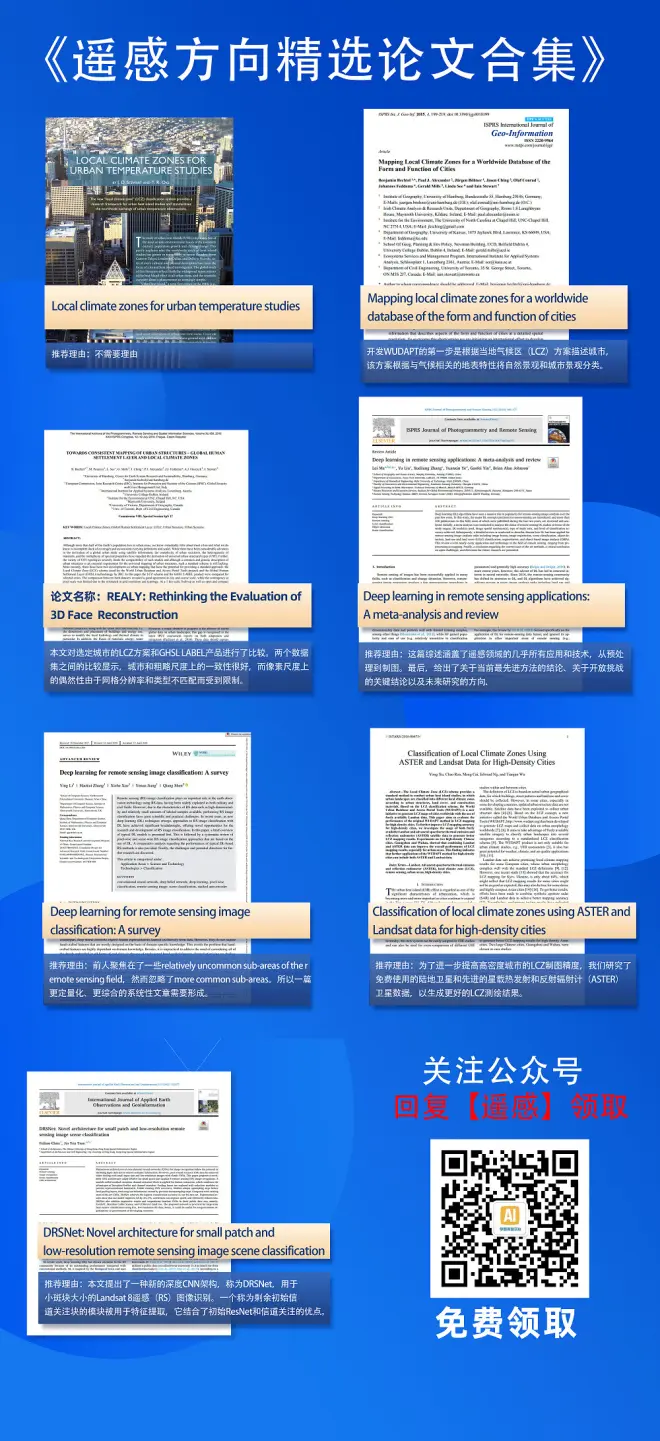
本研究課題適合遙感、規(guī)劃、地理信息系統(tǒng)等學(xué)科領(lǐng)域的學(xué)生與從業(yè)者,以及所有有志于貢獻(xiàn)開源數(shù)據(jù)社區(qū)WUDAPT的人員。
有遙感方向發(fā)論文需求的同學(xué)可以聯(lián)系小享咨詢1V6論文班服務(wù)!
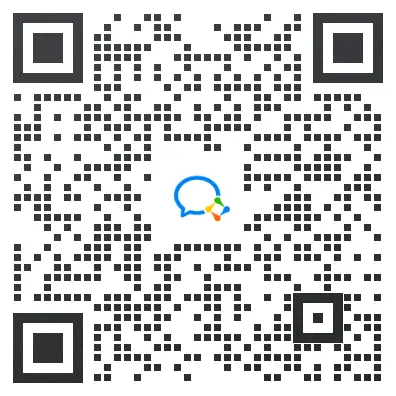