強(qiáng)化學(xué)習(xí)與最優(yōu)控制(英文版)
鏈接:https://pan.baidu.com/s/1-XMmZ0TlS30zlswB0iA5zQ?pwd=my8d?
提取碼:my8d
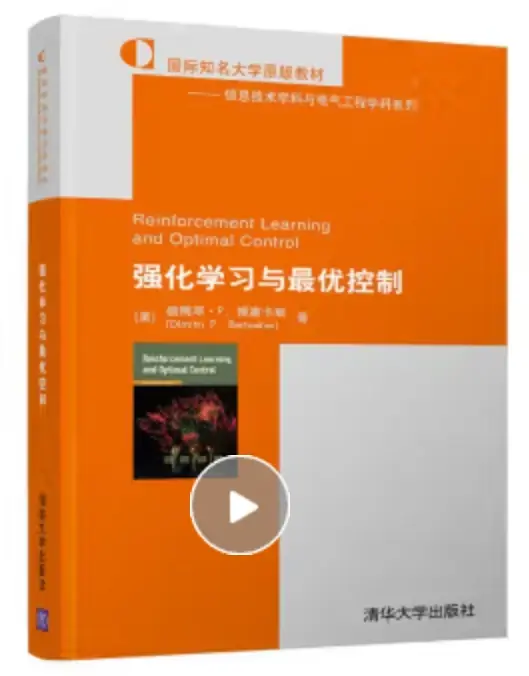
Dimitri P. Bertseka,美國(guó)MIT終身教授,美國(guó)國(guó)家工程院院士,清華大學(xué)復(fù)雜與網(wǎng)絡(luò)化系統(tǒng)研究中心客座教授,電氣工程與計(jì)算機(jī)科學(xué)領(lǐng)域國(guó)際知名作者,著有《非線性規(guī)劃》《網(wǎng)絡(luò)優(yōu)化》《凸優(yōu)化》等十幾本暢銷教材和專著。本書的目的是考慮大型且具有挑戰(zhàn)性的多階段決策問(wèn)題,這些問(wèn)題原則上可以通過(guò)動(dòng)態(tài)規(guī)劃和最優(yōu)控制來(lái)解決,但它們的精確解決方案在計(jì)算上是難以處理的。本書討論依賴于近似的解決方法,以產(chǎn)生具有足夠性能的次優(yōu)策略。這些方法統(tǒng)稱為增強(qiáng)學(xué)習(xí),也可以叫做近似動(dòng)態(tài)規(guī)劃和神經(jīng)動(dòng)態(tài)規(guī)劃等。
本書的主題產(chǎn)生于最優(yōu)控制和人工智能思想的相互作用。本書的目的之一是探索這兩個(gè)領(lǐng)域之間的共同邊界,并架設(shè)一座具有任一領(lǐng)域背景的專業(yè)人士都可以訪問(wèn)的橋梁。
內(nèi)容簡(jiǎn)介
《強(qiáng)化學(xué)習(xí)與最優(yōu)控制(英文版)》的目的是考慮大型且具有挑戰(zhàn)性的多階段決策問(wèn)題,這些問(wèn)題原則上可以通過(guò)動(dòng)態(tài)規(guī)劃和優(yōu)控制來(lái)解決,但它們的解決方案在計(jì)算上是難以處理的?!稄?qiáng)化學(xué)習(xí)與最優(yōu)控制(英文版)》討論依賴于近似的解決方法,以產(chǎn)生具有足夠性能的次優(yōu)策略。這些方法統(tǒng)稱為增強(qiáng)學(xué)習(xí),也可以叫做近似動(dòng)態(tài)規(guī)劃和神經(jīng)動(dòng)態(tài)規(guī)劃等。《強(qiáng)化學(xué)習(xí)與最優(yōu)控制(英文版)》的主題產(chǎn)生于優(yōu)控制和人工智能思想的相互作用?!稄?qiáng)化學(xué)習(xí)與最優(yōu)控制(英文版)》的目的之一是探索這兩個(gè)領(lǐng)域之間的共同邊界,并架設(shè)一座具有任一領(lǐng)域背景的人士都可以訪問(wèn)的橋梁。
作者簡(jiǎn)介
Dimitri P. Bertseka,美國(guó)MIT終身教授,美國(guó)國(guó)家工程院院士,清華大學(xué)復(fù)雜與網(wǎng)絡(luò)化系統(tǒng)研究中心客座教授。電氣工程與計(jì)算機(jī)科學(xué)領(lǐng)域國(guó)際知名作者,著有《非線性規(guī)劃》《網(wǎng)絡(luò)優(yōu)化》《凸優(yōu)化》等十幾本暢銷教材和專著。
目錄
Contents
1. Exact Dynamic Programming
1.1. DeterministicDynamicProgramming . . . . . . . . . . . p. 2
1.1.1. DeterministicProblems . . . . . . . . . . . . . . p. 2
1.1.2. TheDynamicProgrammingAlgorithm . . . . . . . . p. 7
1.1.3. Approximation inValue Space . . . . . . . . . . . p. 12
1.2. StochasticDynamicProgramming . . . . . . . . . . . . . p. 14
1.3. Examples,Variations, and Simplifications . . . . . . . . . p. 18
1.3.1. Deterministic ShortestPathProblems . . . . . . . . p. 19
1.3.2. DiscreteDeterministicOptimization . . . . . . . . . p. 21
1.3.3. Problemswith aTermination State . . . . . . . . . p. 25
1.3.4. Forecasts . . . . . . . . . . . . . . . . . . . . . p. 26
1.3.5. Problems with Uncontrollable State Components . . . p. 29
1.3.6. PartialState Information andBelief States . . . . . . p. 34
1.3.7. LinearQuadraticOptimalControl . . . . . . . . . . p. 38
1.3.8. SystemswithUnknownParameters -Adaptive . . . . . .
Control . . . . . . . . . . . . . . . . . . . . . p. 40
1.4. ReinforcementLearning andOptimalControl - Some . . . . . .
Terminology . . . . . . . . . . . . . . . . . . . . . . p. 43
1.5. Notes and Sources . . . . . . . . . . . . . . . . . . . p. 45
2. Approximation in Value Space
2.1. ApproximationApproaches inReinforcementLearning . . . . p. 50
2.1.1. General Issues ofApproximation inValue Space . . . . p. 54
2.1.2. Off-Line andOn-LineMethods . . . . . . . . . . . p. 56
2.1.3. Model-Based Simplification of the Lookahead . . . . . .
Minimization . . . . . . . . . . . . . . . . . . . p. 57
2.1.4. Model-Free off-Line Q-Factor Approximation . . . . p. 58
2.1.5. Approximation inPolicy Space onTop of . . . . . . . .
ApproximationinValue Space . . . . . . . . . . . p. 61
2.1.6. When is Approximation in Value Space Effective? . . . p. 62
2.2. Multistep Lookahead . . . . . . . . . . . . . . . . . . p. 64
??ii
viii Contents
2.2.1. Multistep Lookahead and Rolling Horizon . . . . . . p. 65
2.2.2. Multistep Lookahead and Deterministic Problems . . . p. 67
2.3. Problem Approximation . . . . . . . . . . . . . . . . . p. 69
2.3.1. Enforced Decomposition . . . . . . . . . . . . . . p. 69
2.3.2. Probabilistic Approximation - Certainty Equivalent . . . .
Control . . . . . . . . . . . . . . . . . . . . . p. 76
2.4. Rollout and the Policy Improvement Principle . . . . . . . p. 83
2.4.1. On-Line Rollout for Deterministic Discrete . . . . . . . .
Optimization . . . . . . . . . . . . . . . . . . . p. 84
2.4.2. Stochastic Rollout and Monte Carlo Tree Search . . . p. 95
2.4.3. Rollout with an Expert . . . . . . . . . . . . . p. 104
2.5. On-Line Rollout for Deterministic Infinite-Spaces Problems - . . .
Optimization Heuristics . . . . . . . . . . . . . . . . p. 106
2.5.1. Model Predictive Control . . . . . . . . . . . . . p. 108
2.5.2. Target Tubes and the Constrained Controllability . . . . .
Condition . . . . . . . . . . . . . . . . . . . p. 115
2.5.3. Variants of Model Predictive Control . . . . . . . p. 118
2.6. Notes and Sources . . . . . . . . . . . . . . . . . . p. 120
3. Parametric Approximation
3.1. Approximation Architectures . . . . . . . . . . . . . . p. 126
3.1.1. Linear and Nonlinear Feature-Based Architectures . . p. 126
3.1.2. Training of Linear and Nonlinear Architectures . . . p. 134
3.1.3. Incremental Gradient and Newton Methods . . . . . p. 135
3.2. Neural Networks . . . . . . . . . . . . . . . . . . . p. 149
3.2.1. Training of Neural Networks . . . . . . . . . . . p. 153
3.2.2. Multilayer and Deep Neural Networks . . . . . . . p. 157
3.3. Sequential Dynamic Programming Approximation . . . . . p. 161
3.4. Q-Factor Parametric Approximation . . . . . . . . . . . p. 162
3.5. Parametric Approximation in Policy Space by . . . . . . . . .
Classification . . . . . . . . . . . . . . . . . . . . . p. 165
3.6. Notes and Sources . . . . . . . . . . . . . . . . . . p. 171
4. Infinite Horizon Dynamic Programming
4.1. An Overview of Infinite Horizon Problems . . . . . . . . p. 174
4.2. Stochastic Shortest Path Problems . . . . . . . . . . . p. 177
4.3. Discounted Problems . . . . . . . . . . . . . . . . . p. 187
4.4. Semi-Markov Discounted Problems . . . . . . . . . . . p. 192
4.5. Asynchronous Distributed Value Iteration . . . . . . . . p. 197
4.6. Policy Iteration . . . . . . . . . . . . . . . . . . . p. 200
4.6.1. Exact Policy Iteration . . . . . . . . . . . . . . p. 200
4.6.2. Optimistic and Multistep Lookahead Policy . . . . . . .
Iteration . . . . . . . . . . . . . . . . . . . . p. 205
4.6.3. Policy Iteration for Q-factors . . . . . . . . . . . p. 208
Contents i??
4.7. Notes and Sources . . . . . . . . . . . . . . . . . . p. 209
4.8. Appendix: MathematicalAnalysis . . . . . . . . . . . p. 211
4.8.1. Proofs for Stochastic ShortestPathProblems . . . . p. 212
4.8.2. Proofs forDiscountedProblems . . . . . . . . . . p. 217
4.8.3. ConvergenceofExact andOptimistic . . . . . . . . . .
Policy Iteration . . . . . . . . . . . . . . . . p. 218
5. Infinite Horizon Reinforcement Learning
5.1. Approximation in Value Space - Performance Bounds . . . p. 222
5.1.1. LimitedLookahead . . . . . . . . . . . . . . . p. 224
5.1.2. Rollout and Approximate Policy Improvement . . . p. 227
5.1.3. ApproximatePolicy Iteration . . . . . . . . . . . p. 232
5.2. FittedValue Iteration . . . . . . . . . . . . . . . . . p. 235
5.3. Simulation-BasedPolicy IterationwithParametric . . . . . . .
Approximation . . . . . . . . . . . . . . . . . . . . p. 239
5.3.1. Self-Learning andActor-CriticMethods . . . . . . p. 239
5.3.2. Model-Based Variant of a Critic-Only Method . . . p. 241
5.3.3. Model-FreeVariant of aCritic-OnlyMethod . . . . p. 243
5.3.4. Implementation Issues ofParametricPolicy . . . . . . .
Iteration . . . . . . . . . . . . . . . . . . . . p. 246
5.3.5. Convergence Issues ofParametricPolicy Iteration - . . . .
Oscillations . . . . . . . . . . . . . . . . . . . p. 249
5.4. Q-Learning . . . . . . . . . . . . . . . . . . . . . p. 253
5.4.1. Optimistic Policy Iteration with Parametric Q-Factor . . .
Approximation- SARSAandDQN . . . . . . . . p. 255
5.5. AdditionalMethods -TemporalDifferences . . . . . . . p. 256
5.6. Exact andApproximateLinearProgramming . . . . . . p. 267
5.7. Approximation inPolicy Space . . . . . . . . . . . . . p. 270
5.7.1. Training byCostOptimization -PolicyGradient, . . . . .
Cross-Entropy,andRandomSearchMethods . . . . p. 276
5.7.2. Expert-BasedSupervisedLearning . . . . . . . . p. 286
5.7.3. ApproximatePolicy Iteration,Rollout, and . . . . . . .
ApproximationinPolicySpace . . . . . . . . . . p. 288
5.8. Notes and Sources . . . . . . . . . . . . . . . . . . p. 293
5.9. Appendix: MathematicalAnalysis . . . . . . . . . . . p. 298
5.9.1. Performance Bounds for Multistep Lookahead . . . . p. 299
5.9.2. Performance Bounds for Rollout . . . . . . . . . . p. 301
5.9.3. Performance Bounds for Approximate Policy . . . . . . .
Iteration . . . . . . . . . . . . . . . . . . . . p. 304
6. Aggregation
6.1. AggregationwithRepresentativeStates . . . . . . . . . p. 308
6.1.1. Continuous State and Control Space Discretization . p. 314
6.1.2. Continuous State Space - POMDP Discretization . . p. 315
?? Contents
6.2. AggregationwithRepresentativeFeatures . . . . . . . . p. 317
6.2.1. Hard Aggregation and Error Bounds . . . . . . . . p. 320
6.2.2. AggregationUsingFeatures . . . . . . . . . . . . p. 322
6.3. Methods for Solving theAggregateProblem . . . . . . . p. 328
6.3.1. Simulation-BasedPolicy Iteration . . . . . . . . . p. 328
6.3.2. Simulation-Based Value Iteration . . . . . . . . . p. 331
6.4. Feature-BasedAggregationwith aNeuralNetwork . . . . p. 332
6.5. BiasedAggregation . . . . . . . . . . . . . . . . . . p. 334
6.6. Notes and Sources . . . . . . . . . . . . . . . . . . p. 337
6.7. Appendix: MathematicalAnalysis . . . . . . . . . . . p. 340
References . . . . . . . . . . . . . . . . . . . . . . . p. 345
Index . . . . . . . . . . . . . . . . . . . . . . . . . . p. 369
查看全部↓